
Owing to the significant cost and time required for wet lab-based AMP screening, researchers have framed the task as an ML problem. This work provides guidance on design choices for CANs that lead to better interpretability for regulatory genomics without sacrificing generalization performance.Īntimicrobial peptides have gained immense attention in recent years due to their potential for developing novel antibacterial medicines, next-generation anti-cancer treatment regimes, etc. GLIFAC robustly uncovers motif interactions across a wide spectrum of model choices. To address this issue, we propose Global Interactions via Filter Activity Correlations (GLIFAC).
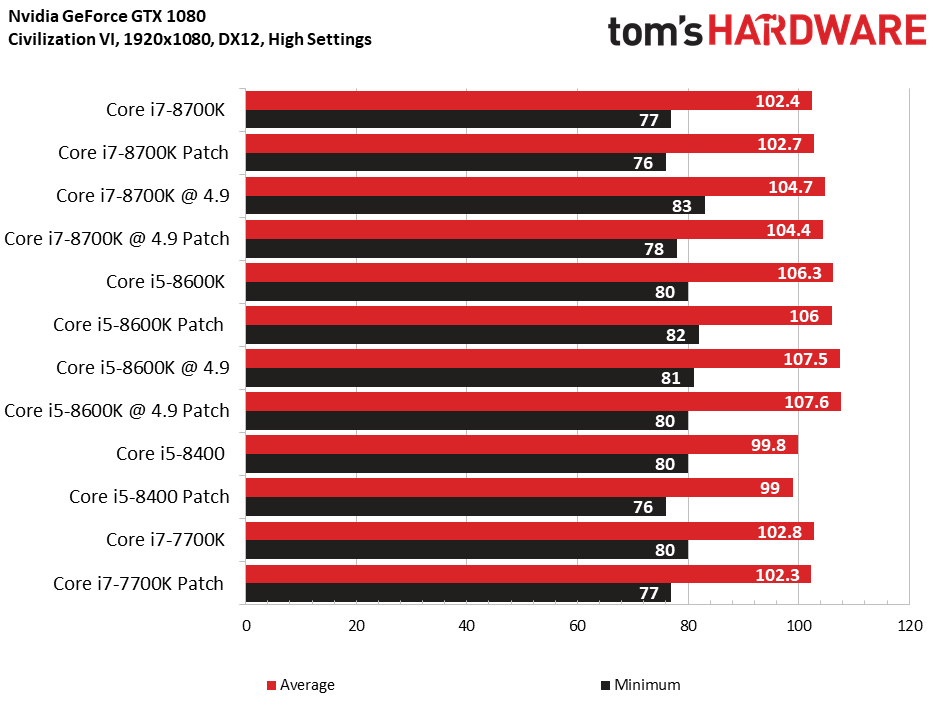
on an individual sequence basis) is noisy, leading to many false positive motif interactions. We find that irrespective of design choice, interpreting local attention (i.e. Here we perform an empirical study on synthetic data to test the efficacy of uncovering motif interactions in CANs. However, it is unclear the extent to which this is true in practice. In principle, convolution-attention networks (CANs) should provide an inductive bias to infer motif interactions convolutions can capture motifs while self-attention learns their interactions. Our proposed method not only demonstrates the interpretability of the ESN readout layer but also provides a computationally inexpensive, unsupervised data-driven approach for identifying uncontrolled variables affecting real-world data from nonlinear dynamical systems.Ī major goal of computational genomics is to understand how sequence patterns, called motifs, interact to regulate gene expression. We demonstrate this approach by extracting the values of three dynamical parameters ($\sigma$, $\rho$, $\beta$) from a dataset of Lorenz systems where all three parameters are varying among different trajectories. We can extract these dynamical parameters by examining the geometry of the readout layer weights through principal component analysis. We show that the parameters governing the dynamics of a complex nonlinear system can be encoded in the learned readout layer of an ESN. However, ESN models are often seen as black-box predictors that lack interpretability.

Reservoir computing architectures known as echo state networks (ESNs) have been shown to have exceptional predictive capabilities when trained on chaotic systems.
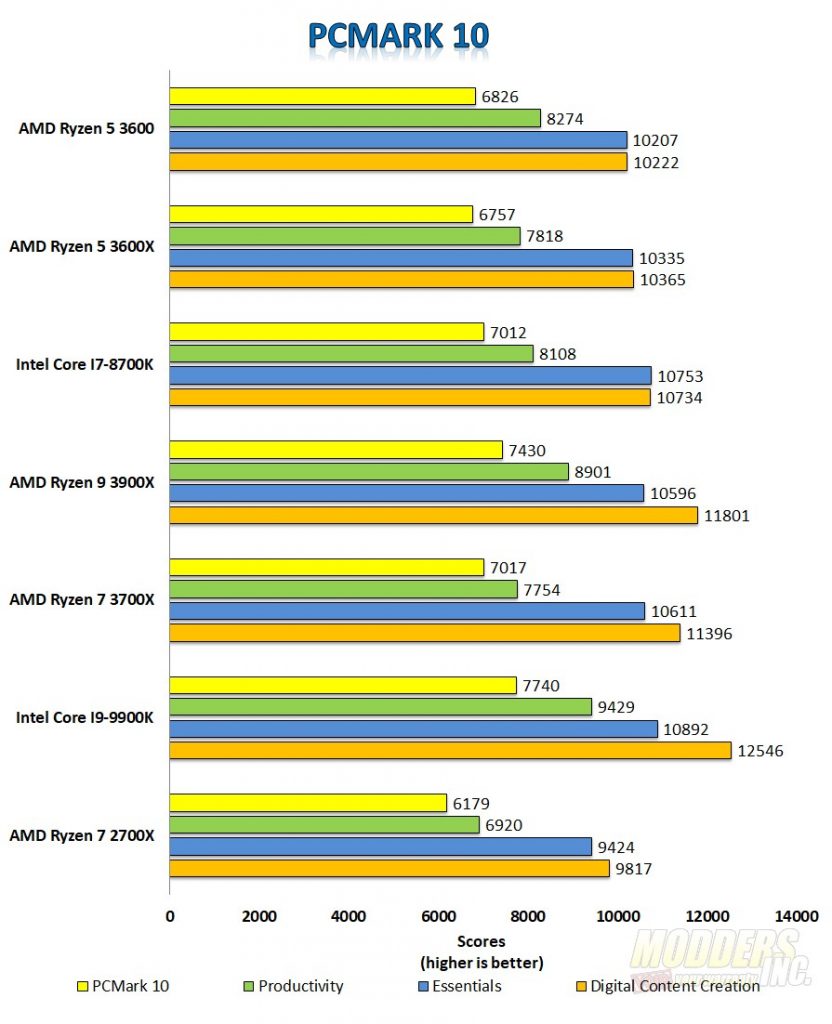
This workshop will fulfill this unmet need and facilitate community building with hundreds of ML researchers beginning projects in this area, the workshop will bring them together to consolidate the fast-growing area of "AI for Science" into a recognized field.
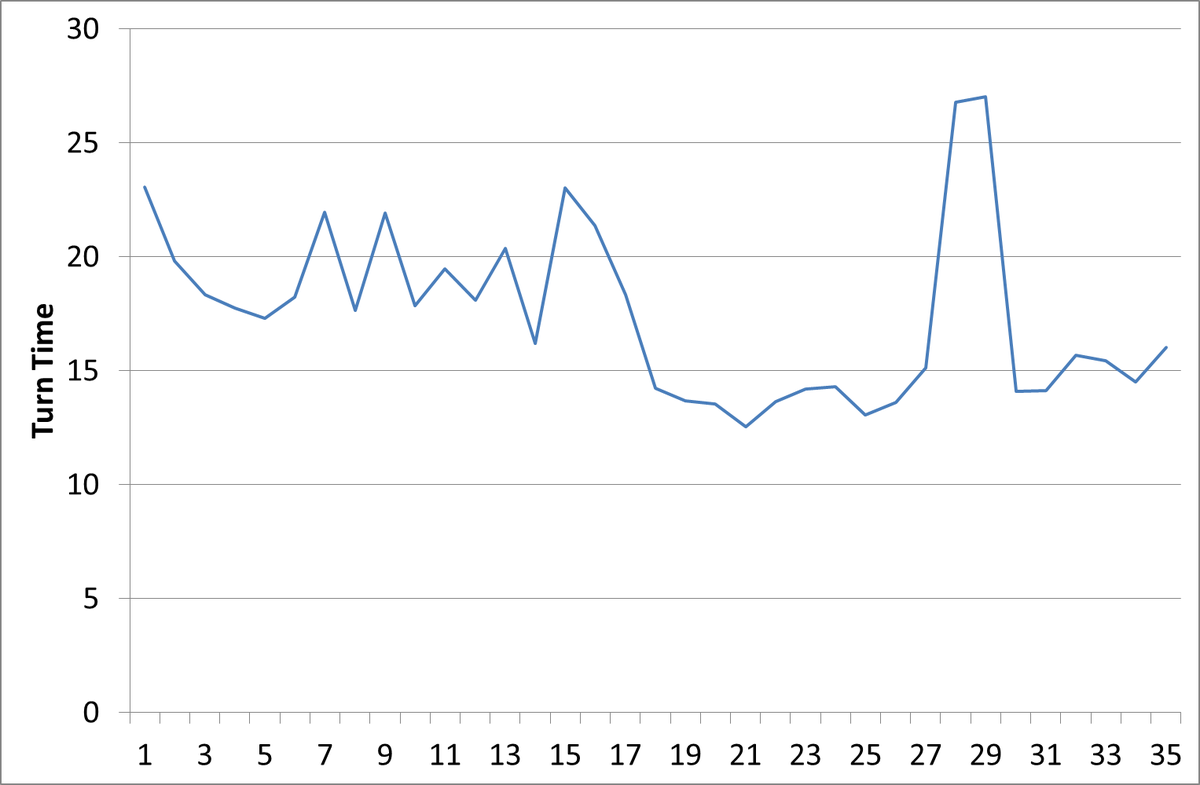
While many workshops focus on AI for specific scientific disciplines, they are all concerned with the methodological advances within a single discipline (e.g., biology) and are thus unable to examine the crucial questions mentioned above. However, very little work has been done to bridge these gaps, mainly because of the missing link between distinct scientific communities. Despite this promise, several critical gaps stifle algorithmic and scientific innovation in "AI for Science": (1) Unrealistic methodological assumptions or directions, (2) Overlooked scientific questions, (3) Limited exploration at the intersections of multiple disciplines, (4) Science of science, (5) Responsible use and development of AI for science. It has solved scientific challenges that were never solved before, e.g., predicting 3D protein structure, imaging black holes, automating drug discovery, and so on. Machine learning (ML) has revolutionized a wide array of scientific disciplines, including chemistry, biology, physics, material science, neuroscience, earth science, cosmology, electronics, mechanical science.
